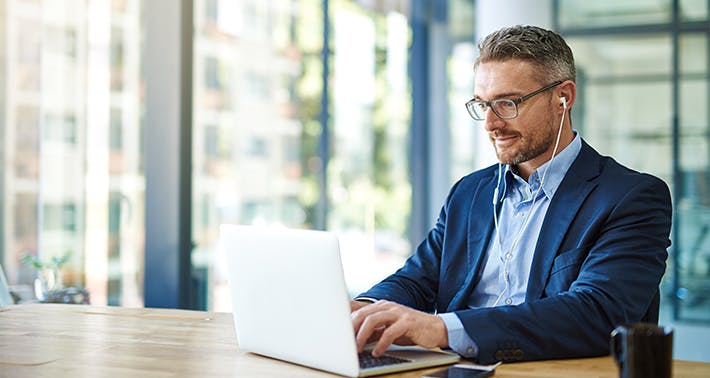
Why Are Explainable AI and Responsible AI Important in the Financial Compliance Industry?

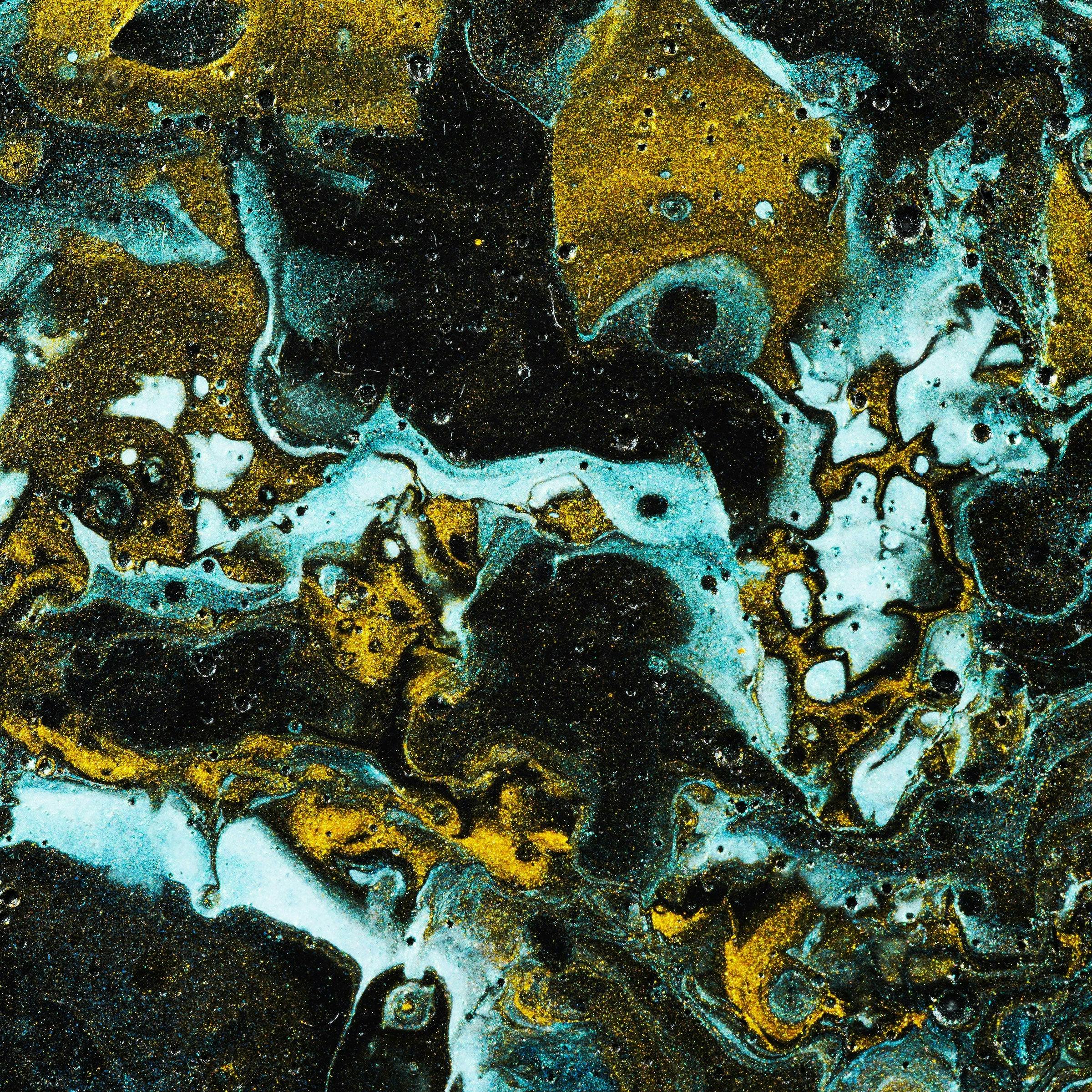
Artificial Intelligence (AI) has transformed industries, enabling smarter decision-making, automation and innovation. However, as AI systems become more complex and widespread, concerns over transparency, fairness and accountability have grown.
Two critical concepts addressing these concerns are explainable AI and responsible AI. While closely related, these frameworks serve distinct but complementary purposes in ensuring ethical and trustworthy AI systems, especially in the financial industry.
What Is Explainable AI?
Explainable AI refers to AI models and systems designed to make their decisions and processes understandable to humans.
Many early AI systems within financial compliance, particularly deep learning models, often operated somewhat as “black boxes,” where their decision-making logic was unclear.
Explainable AI aims to open this black box by providing clear insights into how and why an AI model arrives at a particular conclusion.
Importance of Explainable AI in Financial Compliance
- Regulatory requirements: Financial institutions must comply with laws such as the General Data Protection Regulation (GDPR), EU AI Act and Digital Operational Resilience Act (DORA), which require transparency in AI-driven decision making.
- Fraud detection and risk management: AI is widely used to detect fraudulent activities and assess risks. Explainable AI ensures that flagged transactions or risk scores can be justified and audited.
- Bias mitigation in financial decisions: AI-driven financial decisions, such as mortgage approvals, must be explainable to ensure fairness and prevent discrimination.
Approaches to Explainable AI
Explainable AI uses techniques that make AI models more transparent and interpretable. Approaches to explainable AI can be categorized into intrinsic (built-in explainability) and post-hoc (explanations after model training).
Intrinsic methods involve designing inherently interpretable models, such as decision trees, linear regression, and rule-based systems.
Post-hoc methods can explain complex machine learning models through techniques such as SHAP (Shapley Additive Explanations), LIME (Local Interpretable Model-Agnostic Explanations), and counterfactual explanations, which analyze feature importance or generate alternative scenarios to interpret decisions.
Other approaches for deep learning models include feature visualization, layer-wise relevance propagation, and example-based explanations.
Choosing the right approach depends on factors such as regulatory compliance, user trust, and debugging needs, ensuring AI systems remain transparent and accountable.
Because of their complexity, it’s notoriously hard to get a complete explanation of a decision made by a Large Language Model (“LLM”) within financial compliance, which is why it’s often used as part of a decision tree, or decision-making process, rather than completely standalone.
However, LLMs can be prompted to explain a particular answer, and the new breed of “reasoning” models does mimic the process that a human might use to reach a decision. This, combined with other data, can be used to produce a defensible explanation of why a particular decision has been made.
What Is Responsible AI?
Responsible AI is a broader concept that encompasses ethical principles and governance frameworks to ensure AI systems are fair, safe, accountable and aligned with human values. It involves designing, deploying and managing AI systems in ways that prioritize social good and mitigate harm.
Principles of Responsible AI
- Fairness and bias mitigation: AI should not discriminate against individuals or groups in credit assessments, fraud detection, or trading algorithms.
- Accountability and governance: Financial firms must have clear guidelines, audits and oversight mechanisms for AI use in regulatory compliance.
- Privacy and security: AI systems handling sensitive financial data must comply with Know Your Customer, the required step banks must take to check and confirm their clients’ identities, as well as data protection laws.
- Human-centered decision making: AI should augment human compliance officers rather than replace them, allowing for oversight and manual intervention.
- Regulatory and ethical compliance: Institutions should align AI models with evolving financial regulations to avoid legal penalties and ethical breaches.
The Connection Between Explainable AI and Responsible AI in Finance
Explainable AI is a crucial component of Responsible AI because transparency is necessary for accountability and fairness in financial services. Without understanding how AI reaches its decisions, it’s challenging to assess its ethical and regulatory implications.
A truly responsible AI system integrates explainability as a foundational requirement, ensuring that AI operates with fairness, safety and trustworthiness in financial compliance.
Best Practices for Explainable and Responsible AI in Financial Compliance
Here are five best practices financial institutions can take to help ensure explainable and responsible AI:
- Adopt AI ethics frameworks: Financial institutions should follow industry and governmental AI ethics guidelines to help ensure compliance.
- Use transparent AI models: Integrate Explainable AI techniques into complex models’ flows.
- Perform regular audits: Conduct AI bias and fairness audits to assess and mitigate risks in financial decision making.
- Engage stakeholders: Involve regulators, policymakers and compliance officers in AI development and deployment.
- Prioritize education: Educating partners and customers about AI-driven financial decisions helps build trust and enables informed decision-making.
Verint Communications Analytics
Verint Communications Analytics, the latest addition to Verint Financial Compliance, leverages explainable and responsible AI to enhance compliance in financial markets by using AI-powered language recognition, transcription and speech analytics.
By identifying compliance gaps in voice communications, it helps ensure adherence to regulatory standards while providing transparent insights into communication patterns and potential risks.
The solution also prioritizes privacy and efficiency by using small local AI models, which process data securely without exposing sensitive information. These smaller models not only enhance data privacy (as they are situated behind the customer’s firewall), which means they’re situated next to the customer’s data.
These models are also more energy-efficient, reducing costs and environmental impact, making them a more responsible AI choice.
This combination of transparency, privacy and sustainability ensures that financial organizations can meet compliance requirements while maintaining ethical AI practices.
As AI becomes more integral to financial compliance, ensuring its ethical and responsible deployment is crucial. Explainable AI helps provide transparency and trust, while Responsible AI helps ensure fairness, accountability and regulatory adherence.
By embracing both, financial institutions can create AI systems that align with compliance standards—where AI-driven financial services operate responsibly and transparently.
Find out more by watching our webinar: Finding the Gaps in Voice Communications: A New Approach to Financial Compliance.